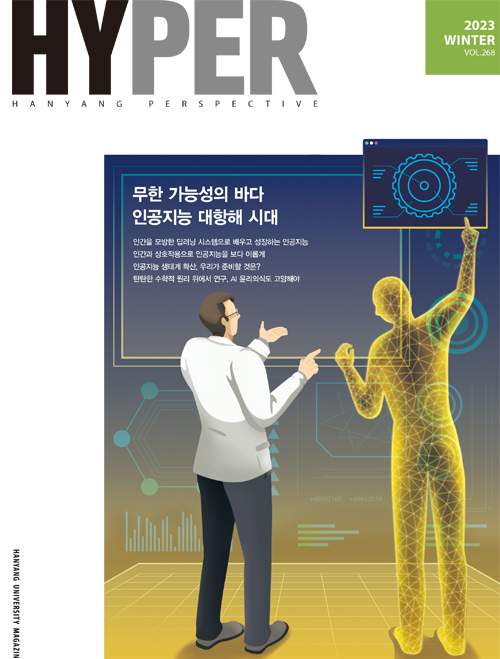
Scroll Down
Artificial intelligence (AI) has been a hot topic for humanity for a long time. In 2023, in particular, interest in AI has been hotter than ever, as an ultra-scale generative AI emerged. Artificial intelligence technology has been used in the past, but it was mainly concentrated in the industry and the academia and did not have much impact on the general public. Now, however, AI has finally made its presence known to the public. We discussed the truths and misunderstandings about artificial intelligence with Professor Yong Suk Choi.
미디어 보도나 사회적 논의는 정확한 사실과 근거를 통해 이뤄져야 한다. 하지만 기존에 본 적 없던 새로운 기술이나 산업, 사회현상 등과 관련해서는 모두가 제대로 이해하고 이야기하기가 어렵다. 그 때문에 조금 더 자극적인 부분에만 초점을 맞추거나 아직은 먼 미래의 얘기가 바로 내일 일어날 것처럼 호도되기도 한다. 인공지능과 관련한 이야기들이 딱 그렇다.
지금은 추억이 된 알파고(AlphaGo). 구글의 딥마인드가 개발한 인공지능 바둑 프로그램 알파고가 2016년 당대 최고 실력자로 꼽힌 이세돌 9단을 이겼을 때, 일부 사람들은 인공지능이 인간을 지배하는 디스토피아를 떠올리며 걱정했다. 하지만 그로부터 7년이 지났어도 우리의 일상은 크게 달라진 것 없이 여전하다. 오픈AI가 2022년 12월 1일 초거대 생성형 AI인 챗GPT를 선보인 이후, 또다시 일부 사람들의 우려와 기대가 커지고 있다. 이쯤 되면, 인공지능에 의한 디스토피아 강림설이 유행 패션처럼 몇 년에 한 번씩 돌고 도는 게 아닌지 의심스럽다.
“일반 대중에게 인기 있는 이슈일수록 과장돼 확산되는 측면이 있습니다. 자율주행차가 처음 선보였을 때도 곧 완전자율주행차가 일상화되어 거리를 활보할 것처럼 이야기됐지만, 현실과 많이 다르죠. 인공지능 분야도 그렇습니다.”
최용석 교수는 ‘인공지능연구실(AI Lab)’을 이끌며 딥러닝 구조 및 최적화, 대규모언어모델(LLM) 기반 자연어 처리, SNS 텍스트 분석 및 시각화, 이미지 및 동영상 처리, 멀티모달 AI 등 인공지능 분야의 다양한 연구를 수행하고 있다. ‘변호사 중계 사이트 질의응답을 위한 대화형 챗봇 기술’ 등 여러 건의 개발 기술 이전에도 성공했다. 딥러닝 모델 기반 대규모 SNS 분석을 통해 코로나 시기에 사람들이 느끼는 불안 감정과 코로나19 확진자 수 변화 추이의 상관관계를 밝혀내 국제 저명학술지에 발표하기도 했다. 최근에는 텍스트 문서의 요약 및 의미론적 이해를 위한 새로운 원천 기술을 개발하고, 이를 자연어 처리 분야의 탑티어 콘퍼런스인 ACL 2023 및 EMNLP 2021, 2023 등에 연이어 발표함으로써 관련 연구에서 세계적 수준의 성과를 입증한 바 있다. 이처럼 인공지능 연구의 최전선에 서 있는 최용석 교수는 인공지능을 조금 더 현실적으로 바라봐야 한다고 지적했다.
“어느 학문 분야든지 그것을 정통으로 연구하는 사람들은 조금 더 보수적이고 조심스러운 입장에서 그 분야의 미래를 예측하는 경향이 있어요. 목표 달성을 위한 기술 발전 단계를 하나하나 넘어가는 것이 얼마나 어려운지 잘 알기 때문입니다. 그런 측면에서 볼 때, 요즘 일부 언론에서 그리는 인공지능 기술의 미래는 너무 성급한 측면이 있습니다.”
Media reports and social discussions should be based on accurate facts and evidence. However, not everyone can properly understand and talk about new technologies, industries, and social phenomena that have never been seen before. For this reason, they tend to focus on the more provocative aspects, or mislead people into thinking that what they thought would not happen for a long time will happen at any moment. This is very true for stories related to artificial intelligence.
AlphaGo, which has now become a memory, was an AI Go program developed by Google that defeated Lee Sedol, one of the best player of the time in 2016. At that time, some people worried about a dystopia where artificial intelligence would dominate humans. However, seven years have passed since then, and our lives have not changed much.
When OpenAI introduced Chat GPT, a super-generative AI chatbot, on December 1, 2022, people’s concerns and expectations about AI started to grow again. At this point, it seems that stories of the advent of dystopia by created by AI re-emerge every few years, like a fashion trend.
“When an issue is popular with the general public, it tends to get exaggerated. When autonomous vehicles were first introduced, people thought fully self-driving cars would soon dominate the streets, but that is far from reality. The same goes for the field of artificial intelligence,” remarked Professor Yong Suk Choi. Prof. Choi, who leads the AI Lab and conducts research in various fields of artificial intelligence – such as deep learning structuring and optimization; large language model (LLM)-based natural language processing; analysis and visualization of social media texts; and multi-modal AI. He has also succeeded in transferring several technologies, including “interactive chatbot technology for answering questions on lawyer service sites.” Moreover, he found a correlation between the anxieties of people during COVID and the trend of changes in the number of confirmed COVID-19 cases, through a deep learning model-based social media analysis, and published the results in a renowned international academic journal.
Recently, he developed a new source technology for summarizing and semantically understanding texts, and presented it at ACL 2023, and EMNLP 2021 and 2023 – top-tier conferences in the field of natural language processing – thereby demonstrating worldclass achievements in related research. Professor Yong Suk Choi, who is at the forefront of artificial intelligence research, pointed out that AI should be viewed more realistically:
“In any academic field, those who study it orthodoxly tend to be more conservative and cautious in predicting the future of that field. That is because they know how hard it is to move through each step of technological development to achieve their goals. From that perspective, the future of artificial intelligence technology is too hastily portrayed in some media.”
제한적이긴 하지만 인공지능 기술은 이미 우리 생활 속에 들어와 있다. 스마트폰, 컴퓨터 등 여러 스마트 기기와 연동돼 우리가 더 쉽게 검색하고 조작하고 활용하도록 돕는다. 인공지능의 성장은 GPU(그래픽 처리를 위한 고성능 장치)와 같이 대용량 정보 처리가 가능한 하드웨어의 발전과 딥러닝 알고리즘과 같은 소프트웨어의 발전이 시너지를 이뤄낸 성과다. 기계학습의 일종인 딥러닝은 뇌 신경망으로 복잡하게 구성된 인간의 두뇌 작동 방식을 흉내 낸 것이다. 최용석 교수도 고성능 딥러닝 모델 개발을 위한 연구를 수행하고 있다.
“과거의 기계학습은 실세계에 존재하는 객체 및 그들 간의 관계를 수학 및 논리학 기반으로 모델링(symbolic 기법)한 뒤 예측, 진단, 추론 등을 수행하게 했습니다. 지식(규칙)기반 시스템, 전문가 시스템, 온톨로지 시스템, 베이지안 네트워크 등이 여기에 해당합니다. 최근에는 인간의 두뇌를 소프트웨어적으로 모방한 딥러닝 모델(sub-symbolic 기법)을 활발하게 활용하고 있죠. 실세계 객체 특성 데이터를 딥러닝 모델의 입출력으로 연결(anchoring)하고, 인간의 두뇌와 비슷하게 딥러닝 모델 내의 연결 가중치를 반복적으로 조정하여 학습시키는 방식입니다. 딥러닝을 통해 인공지능은 더 효율적으로, 거대 규모의 정보를 학습하게 됐습니다.”
딥러닝 시스템을 활용해 특정 작업에서 구할 수 있는 양질의 데이터를 충분히 학습시키면 인공지능은 인간에 근접하거나 가끔은 인간을 뛰어넘는 성과를 보여준다. 최용석 교수는 영상의학 판독 작업이나 대규모 법률 판례 분석, 문서 요약, 특정 주제 보고서 작성, 보험 계리, 약 조제, 생산공정 최적화 및 자동화 등과 같이 정형화되고 반복적이며 세밀한 작업의 경우 인공지능이 보조 또는 대체해 가기가 상대적으로 수월한 편이라고 설명했다. 하지만 이 또한 시간이 필요한 일이다. 현재까지의 딥러닝 모델은 예측/진단/추론 과정의 논리적 설명이 어려울 수 있고, 중대한 작업에서 드물지만 치명적 오류나 재앙적인 결과를 낳을 수 있으므로 전면적인 실용화가 어려운 분야들이 적지 않다. 양질의 대규모 학습 데이터를 구하는 것 자체가 어렵거나 비용적으로 거의 불가능한 경우도 많다. 이와 같이 여러 가지 이유로, 인간처럼 모든 작업에 대해서 완전히 스스로 학습하는 인공지능은 아직도 요원한 것이 사실이다.
Although limited, artificial intelligence technology is already part of our daily lives. It is linked to various smart devices such as smartphones and computers, helping us search and utilize them more easily. The growth of AI is the synergetic result of advances in hardware, capable of processing large amount of information, such as GPUs (highperformance devices for processing graphics), and the development of software, such as deep learning algorithm. Deep learning, a type of machine learning, mimics the way the human brain works, which is complexly composed of brain neural networks. Professor Choi is also conducting research into developing high-performance deep learning models:
“In the past, machine learning modeled objects in the real world and the relationships among them based on mathematics and logic (symbolic techniques), and then made prediction, diagnosis, and deduction. These include knowledge (rule)-based systems, expert systems, ontology systems, and Bayesian networks. These days, deep learning models that mimic the human brain in software (sub-symbolic techniques) are being actively used. This is a method that anchors real world object characteristics data to the input and output of a deep learning model and trains it by repeatedly adjusting the anchored weight within the deep learning model, similar to the human brain. Through deep learning, artificial intelligence can learn large amounts of information more efficiently.”
When a deep learning system is used to learn enough high-quality data available for a specific task, artificial intelligence shows results that are similar to, or sometimes exceeding, those of humans. Professor Choi explained that artificial intelligence can easily assist or replace standardized, repetitive, and detailed tasks such as radiology analysis, analysis of large-scale legal precedents, document summarization, writing reports on specific topics, actuarial work, medication dispensing, and production process optimization and automation. However, this will take time. There are many fields in which practical, full-scale use of deep learning models to date is difficult because the logical explanations of the prediction, diagnosis, and deduction process can be abstruse; and in rare cases, it can produce fatal errors or disastrous results in important tasks. It is also difficult or too costly to obtain large-scale, high-quality learning data in many cases. For all these reasons, would be true to say that AI that completely learn all tasks on their own, like humans, are still a long way off.
The rapid development of artificial intelligence is the result of a synergistic effect of the complementary development of computer hardware and software. In addition, through deep learning, AI can learn large amounts of information more efficiently.
전 세계가 인공지능에 주목하는 요즘 멀티모달 딥러닝 모델(CLIP, DALL-E 등), 대규모언어모델(BERT, BART, GPT 등), 생성형 AI, 설명가능한 AI(XAI) 등 다양한 분야의 인공지능 연구가 이뤄지고 있다. 인공지능의 할루시네이션(AI Hallucination, AI가 사실이 아닌 것을 사실인 양 허위 정보를 생성하는 현상)이나 사회적 편견 표출(Social Bias) 문제에 대해서도 최신 연구가 진행 중이다. 현재 우리나라에서도 해당 분야의 탑티어 콘퍼런스 및 국제저명학술지에 발표되는 연구 결과물이 빠른 속도로 늘어나고 있다. 다만, 미래 인공지능 기술의 바탕이 될 수 있는 원천 이론 및 기술, 고유 AI 모델 개발 면에서 세계 최고 수준과의 격차가 존재하는 것도 사실이다. 아직은 국내의 많은 연구가 딥러닝 응용이나 기존 모델의 튜닝을 통한 성능 향상에 초점이 맞춰져 있는 점도 아쉽다.
“인공지능 분야는 자고 일어나면 읽어야 할 새로운 논문이 넘쳐난다 말할 정도로 기술 발전이 빠릅니다. 2022년 11월까지만 해도 챗GPT는 일반인에게 소개되지 않았어요. 인공지능 분야를 선도하기 위해서는 미래를 내다보는 혜안과 이에 발맞춘 혁신적인 연구가 필요합니다.”
최용석 교수는 그동안의 연구 결과를 지속적으로 고도화하는 한편, ‘보편적 딥러닝(Universal Deep Learning)’ 모델 개발을 위해 노력할 방침이다. 수없이 다양한 문제에 대해 일관된 성능의 해결능력을 갖추고, 나아가 듣도 보도 못한 완전히 새로운 문제에 대해서도 그럴싸한 창발적 해결능력을 보여주는 인공지능. 즉 실제 인간의 일반지능(General Intelligence)에 견줄만한 인공지능을 개발하는 일은 최용석 교수와 수많은 관련 연구자가 염원하는 궁극적인 목표다.
포크레인 한 대가 삽을 든 사람 100명을 대신한 지 오래됐지만 세상은 더 불행해지지 않았다. 삽질을 하던 사람 중 일부는 포크레인이나 스마트폰을 만들고, 일부는 엔터테인먼트 등 더 부가가치가 높은 신산업에 종사하게 됐다. 인류 현대 문명의 중대한 기반이 되는 ‘전자기력’의 개념을 이해하기 시작한 것도 불과 300년 정도밖에 되지 않았다. 최용석 교수는 이 광활한 우주의 시공간에서 한 점으로 표현하기도 힘든 지점에 살고있는 인류가 아직도 모르는 것이 얼마나 많을지는 짐작할 수 없을 정도라고 강조했다.
“언젠가 인간의 지능 발현에 대한 비밀을 과학적으로 더 명확히 규명할 수 있게 된다면, 미래의 인공지능은 지금과는 전혀 다른 수준의 혁신을 이뤄갈 수 있겠죠. 이를 준비하기 위해서라도 기초과학 이론에 대한 기본기를 탄탄히 갖추고, 고전 AI 모델뿐 아니라 개별 작업에 최적화된 최신 딥러닝 모델의 특성을 면밀히 이해‧분석하는 게 중요할 것입니다. 아울러 이들의 특장점을 결합해 시너지 효과를 얻음으로써 보다 범용적인 AI 모델에 대한 연구를 수행하는 것도 향후 인공지능 시대를 주도적으로, 또 혁신적으로 이끌어가는 방향 중 하나라고 생각합니다.”
Today, the whole world is paying attention to artificial intelligence. AI research is being conducted in various fields, including multimodal deep learning models (CLIP, DALL-E, etc.), large language models (BERT, BART, GPT, etc.), generative AI, and eXplainable AI (XAI).
Research is also in progress on the issues of AI Hallucination (a phenomenon in which an AI generates false information) and societal AI bias. Currently, the number of research results published at top-tier conferences, and by internationally renowned academic journals in the field is increasing rapidly in Korea. However, it is also true that there is a gap between the world’s best in terms of original theory and technology that can serve as the basis for future artificial intelligence technology, as well as in unique AI development. It is also unfortunate that much of the domestic research is still focused on deep learning applications and improving performances through tuning of existing models.
“Technology is developing so quickly in the field of artificial intelligence that papers pile up for you to read every morning. ChatGPT was not introduced to the general public until November 2022. To lead the field of artificial intelligence, we need insight into the future and conduct innovative research for it.”
Professor Yong Suk Choi plans to continue to advance his research results and strive to develop a “universal deep learning” model. Artificial intelligence has the ability to solve countless and diverse problems with consistent performance, and demonstrates plausible problem-solving ability for things that had never been heard of before. Therefore, developing AI comparable to human general intelligence is the ultimate goal for Professor Yong Suk Choi and many other researchers like him.
It has been a long time since a forklift replaced 100 people with shovels, but the world did not become unhappier as a result. People who worked with shovels now make forklifts or smartphones, or work in new industries with higher added value, such as entertainment. It has only been about 300 years since people began to understand the concept of “electromagnetic force,” which is an important foundation for modern human civilization. Professor Yong Suk Choi emphasized that it is impossible to guess how much we humans still do not know, living in a space that is merely a dot in this vast space and time of the universe:
“One day, if we can scientifically identify the secrets to human intelligence more clearly, future artificial intelligence will be able to achieve another level of innovation. To prepare for this, it is important to have a solid foundation in basic scientific theory, and to thoroughly understand and analyze the characteristics of not only classical AI models, but also the latest deep learning models optimized for individual tasks. In addition, I believe that conducting research on more general-purpose AI models by combining their outstanding points and achieving synergy effects is one of the ways to lead the AI era proactively and innovatively in the future.”